Md Ashad Alam, PhD
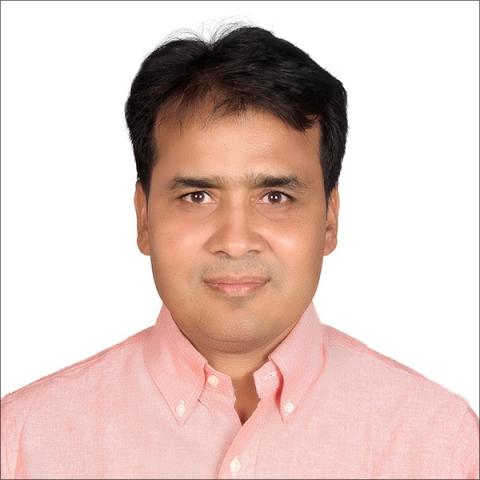
Education & Affiliations
Areas of Expertise
Biography
Dr. Md Ashad Alam is currently an Instructor of Statistics and Data Science in the John W. Deming Department of Medicine - Division of Biomedical Informatics and Genomics, where he serves as a bridge between teaching and research. He achieved his Bachelor of Science and Master of Science degrees in Statistics from the Department of Statistics at the University of Rajshahi, Bangladesh. He went on to receive a Ph.D. in Statistical Science from the Institute of Statistical Mathematics in Japan, where he focused on the study of statistical machine learning. Dr. Alam completed two postdoctoral fellowships at Tulane before his faculty recruitment: first in the Department of Biomedical Engineering in the School of Science and Engineering, then in the Department of Global Biostatistics and Data Science in the School of Public Health and Tropical Medicine.He has served as a Lecturer, Assistant Professor, Associate Professor, and Professor in the Department of Statistics at Hajee Mohammad Danesh Sciences & Technology University.
Dr. Alam’s research interests are theoretical and computational aspects of statistical machine learning, representation learning, combinatorial algorithms, robust statistics, and their applications to multi-view biomedical data analysis. His work is published in several peer-reviewed journals, including Briefing in Bioinformatics, Neurocomputing, Computational Statistics and Data Analysis, Journal of Neuroscience Methods, and PLOS one. He is also a member of Tulane University’s Center for Biomedical Informatics and Genomics and the American Statistical Association (ASA).
Courses
Research
Dr. Alam’s research focuses on the integrated analysis of multi-view data in biomedical applications, including projects which aim to reveal new fundamental biological insights of complex diseases and those centered on more clinical applications.
Statistical machine learning: dimensionality reduction, feature selection, non-parametric models, and inference methods for integrative analyses of multi-omics biomedical data including genome, epigenome, transcriptome, proteome, metabolome, lipidome, and medical image of complex diseases.
Deep learning: the advances in deep learning technologies provide new effective paradigms to obtain end-to-end learning models from complex, high-dimensional and heterogeneous biomedical data. Deep learning approaches could be a vehicle for translating big biomedical data into improved human health.
Robust statistical and adversarial machine learning: non-parametric inference, robustness of linear multivariate approaches, robustness of kernel methods, and incorporate as well as invent adversarial machine learning techniques for given complex diseases prediction using a multi-view biomedical dataset.
As a statistical scientist, Dr. Alam’s research interests are in the areas of theoretical and computational aspects of data science, including statistical machine learning, deep learning, robust statistics, and adversarial machine learning.